Segmentation matters more than ever
As messages multiply, yours must fit customer best
- |
- Written by Steven Ramirez
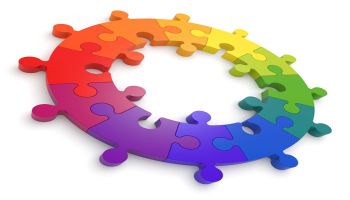
We’re all besieged by a deluge of marketing messages, many of which land with a thud.
Information that helps us to research a key purchase, evaluate options, and identify the best deal is rare—but valuable.
What can your bank do to present such highly relevant information in a way that your customers will value?
Segmentation is a widely used technique in marketing, a way of dividing customers into designated groups based on key attributes, such as demographics, attitudes, or buying behaviors. Customer segmentation enables you to target your messages with more precision.
Marketers typically use demographics, geography, and lifestyle profiles to create basic customer segments. In companies with more mature marketing practices, they may also use data reflecting customer profitability or cost to service the relationship. These fundamental approaches are helpful, but aren’t robust enough to enable you to deliver the right message, to the right customer, at the right time, using the right channel.
Let the data do the talking
Organizations that successfully use segmentation strategies strike a difficult balance.
On one hand, their efforts are highly scalable, sending communications to large numbers of people.
However, they make each prospect or customer feel like the message has been tailored to meet their individual needs.
One way to achieve this balance is by applying predictive analytics techniques to improve customer segmentation. This ensures that each customer receives a relevant offer that resonates.
Traditional market researchers use surveys to segment customers, and survey analysis helps to uncover groups of customers with similar characteristics who may respond to similar messaging. However, surveys are based on what people say they will do—not necessarily what they actually will do. By focusing on behavior, and including information on transactions, data mining helps to increase marketing effectiveness.
On a customer-by-customer basis, data mining reveals which offers to make and helps to explain why. Using predictive analytics, institutions can align each one of their customers into a distinct segment and develop unique messages tailored specifically to meet customer needs, as well as key business objectives.
The result is a win-win scenario—customers are offered the most relevant products and services, leading to more profitable relationships for the company.
Customer segmentation in action
While data mining and predictive analytics can significantly improve the effectiveness of customer segmentation, there is more than one approach you can take. “Value-based” and “vulnerability” segmentation represent two of the most successful methods organizations can employ. Each technique meets a specific marketing need.
Value-based Segmentation: Which customers are most valuable?
To maximize your profitability, you can use analytics to identify your best prospects for cross-sell and upsell offers.
Value can be measured based on a customer’s total revenue generated over the last year. You can use proxies for revenues, or profitability, if you can’t track the actual contribution of each product or account.
Then, customers can be ranked from highest value to lowest and organized into ten subgroups. (This is known as “deciling.”) The customers in the highest deciles should receive your greatest focus and attention. You could also run an analysis like this at a household level, if your bank is able to capture that data.
Vulnerability Segmentation: Which customers are most likely to leave?
Customers can also be segmented in order to better prevent the loss of profitable customers to churn. As it is almost always more expensive to acquire a new customer than to keep a current one, banks can benefit from a data-driven approach to retention.
By segmenting customers based on vulnerability, or likelihood to leave, organizations can send customized offers to the people most at-risk of departing. Advanced analytics can enable you to be more proactive, knowing who to reach out to.
You can assess the risk of churn on a monthly basis, and develop automated processes to alert you when high-value customers begin to exhibit behaviors that indicate they may be preparing to leave.
For example, your predictive modeling might indicate that declining balances, dramatically reduced transaction volumes, elimination of direct deposit, and other key factors are early warning signs that the relationship is in trouble.
In order to truly gain value from customer segmentation and data mining, companies need to be ready to take swift and decisive action based on their findings. That’s why it is essential to start thinking about deployment from the very beginning. When starting a segmentation project, one of the first questions to ask is, “What would an ideal solution look like and how would it be deployed?” Business understanding, which should always be the first phase of a data mining project, is crucial as it connects the mathematics to the real problem at hand.
Ultimately, the bank’s business issues, and its strategy and operating model, should shape what questions to ask of the data. Without this clarity and alignment, the analysis is unlikely to reveal insights that you can act on. Segmentation based on analyzing customer behavior can boost the effectiveness of your marketing efforts when strategy and analytics are well-integrated.
Tagged under Retail Banking, Customers,